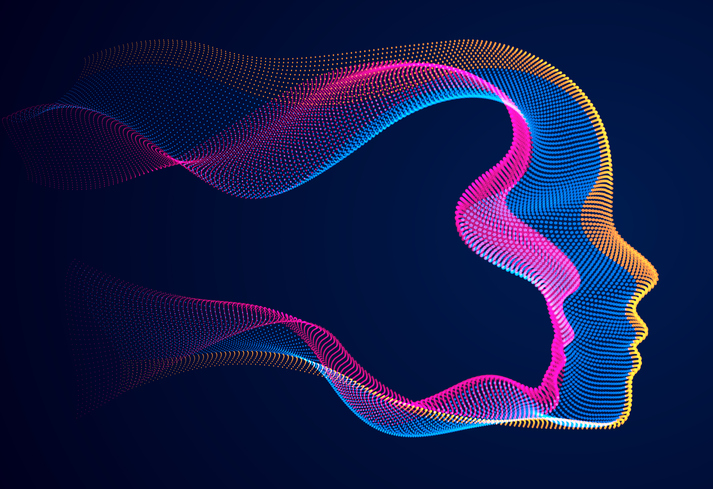
5 methods AI will influence scientific trials this yr
Whereas 2024 might not eradicate the dearth of illustration in scientific trials, will probably be a pivotal yr through which important progress is made because of the combination of AI. Healthcare leaders have an unprecedented alternative to leverage the potential of AI to handle healthcare disparities, particularly in scientific trials. Right here we discover 5 methods AI will rework scientific trials.
- Establish underrepresented populations
Scientific analysis usually fails to replicate various populations, resulting in an incomplete understanding of remedy effectiveness. A US research of greater than 3,000 sufferers enrolled in most cancers trials discovered that black and Hispanic sufferers had decrease Part I enrollment. The underrepresentation of sure teams in scientific trials dangers overlooking variations in drug metabolism, aspect impact profiles and outcomes. This omission can result in dangerous responses to therapies and an incomplete understanding of remedy effectiveness.
AI can play a vital function in figuring out underrepresented populations in scientific trials by rapidly analyzing giant quantities of current healthcare information. Utilizing machine studying (ML) and AI, researchers can achieve perception into affected person demographics, genetic profiles, and different healthcare information to know and tackle the underrepresentation of particular populations. This data can information researchers and trial organizers in actively focusing on and interesting particular demographic teams that will have been ignored or underrepresented previously.
- Optimize trial design and website choice
Deciding on the fitting location, eradicating boundaries to participation, projecting correct enrollment numbers, and sustaining constant communication between principal investigators (PIs) and contributors are all vital to the success of a research. AI optimizes all these processes to make sure trial protocols, eligibility standards, and recruitment efforts are extra inclusive from the beginning.
By analyzing historic analysis information and taking demographic elements under consideration, AI may help researchers establish ideally suited analysis websites and PIs/scientific analysis organizations (CROs). AI also can assist establish group analysis websites that preserve trusted relationships with sufferers who are sometimes ignored throughout the litigation course of.
Moreover, AI might be leveraged to establish the potential boundaries to participation for various sufferers, and AI-powered units may help shut the gaps. For instance, based on a report from Deloitte Insights, entry is the highest barrier to participation in varied scientific trials. AI-powered wearable units function a transformative answer by minimizing the necessity for contributors to bodily journey to trial websites. This improves accessibility for people who wish to take part in these research, enhancing recruitment and participation of various affected person populations.
- Increase affected person engagement and recruitment methods
Recruiting sufferers is commonly a significant bottleneck in scientific trials, requiring important time and sources. In truth, as much as 29% of section III trials fail because of poor recruitment methods. AI can pace up these processes, predict affected person availability primarily based on historic information, and establish and scale back biases in trial recruitment processes to make efforts extra profitable.
AI-powered algorithms can rapidly analyze a variety of things past demographic and well being information (together with socioeconomic standing, cultural background, and geographic location) to establish ideally suited scientific trial contributors. These insights enhance decision-making and permit researchers to design extra inclusive recruitment methods primarily based on varied elements.
Main pharmaceutical firms reminiscent of Amgen, Bayer and Novartis are on the forefront of leveraging AI. They’re actively coaching AI techniques to research large information units, together with billions of public well being information, prescription information, and medical health insurance claims. This method not solely streamlines the identification of potential trial sufferers, however in some circumstances has additionally lower enrollment time in half.
Moreover, the facility of AI may help ship transformative, person-centered care. GenAI-based insights assist physicians develop tailor-made suggestions on the “subsequent finest motion” – one of the best ways to interact totally different affected person populations in a culturally related approach.
- Allow real-time monitoring and adaptive testing
AI permits real-time monitoring of trial contributors by way of wearable units and sensors, permitting rapid identification of any variations or biases that will come up throughout the trial.
AI instruments will also be used to observe website efficiency as soon as the trial has begun, detect uncomfortable side effects and predict outcomes, permitting researchers to establish potential issues or traits early within the course of. One research discovered that ML prediction fashions decreased most cancers mortality by 15-25% in a number of scientific trials, and in addition discovered proof that ML algorithms help the early detection and prognosis of illnesses, enhancing total trial success.
This synchronous suggestions loop improves the effectivity and efficacy of research by enabling adaptive research design, the place protocols might be adjusted to handle points, guarantee equal illustration of contributors, prioritize affected person security, and enhance total improvement success of recent therapies.
- Fight biases in information assortment
Within the context of healthcare information and scientific trials, lowering bias is essential to making sure the effectiveness, equity and security of medical therapies. AI has the potential to eradicate long-standing biases in healthcare information, particularly in digital well being information (EMR) and digital well being information (EHR).
When AI techniques are correctly applied and skilled, biases might be averted and information assortment strategies might be improved to make sure various populations are precisely represented. One of many key challenges is the dearth of range in scientific datasets, which may result in biased AI algorithms. If the coaching information misrepresents the inhabitants, AI is susceptible to amplifying biases, probably resulting in undesirable outcomes or misdiagnoses. To deal with this, AI can synthesize underrepresented information and detect biases within the information assortment and preparation phases, creating know-how that’s fairer and extra correct. Moreover, involving clinicians in information science groups achieves a broader perspective and might forestall biases at totally different phases of algorithm improvement and monitoring.
The (considerably bumpy) highway to success
The combination of AI applied sciences holds promise for growing outreach efforts, streamlining recruitment processes, and addressing long-standing boundaries and biases that hinder range and inclusion in scientific trials. But there are boundaries to their efficient implementation, together with resistance to alter or distrust, safety considerations, excessive prices of creating customized techniques, correct utilization pointers, and workers coaching.
The most important problem delaying widespread adoption and success is enhancing the breadth, high quality, range, and accessibility of the underlying information on which these AI techniques are skilled. With out addressing this head-on, we are going to proceed to see biases persist and hallucinations happening that comprise false or deceptive data.
There are a variety of promising federal efforts underway to assist information our path, such because the FDA's steerage round range motion plans for scientific trials, the President's govt order on using AI, the FDA's plans to launch a Digital Well being Advisory Committee, and the EU AI legislation. It will likely be vital for leaders to align using AI with these rising laws. By taking the fitting steps, it’s attainable to create AI techniques that profit everybody and can positively rework scientific trial processes, in the end serving to to scale back healthcare inequities.
Photograph: Sylverarts, Getty Pictures