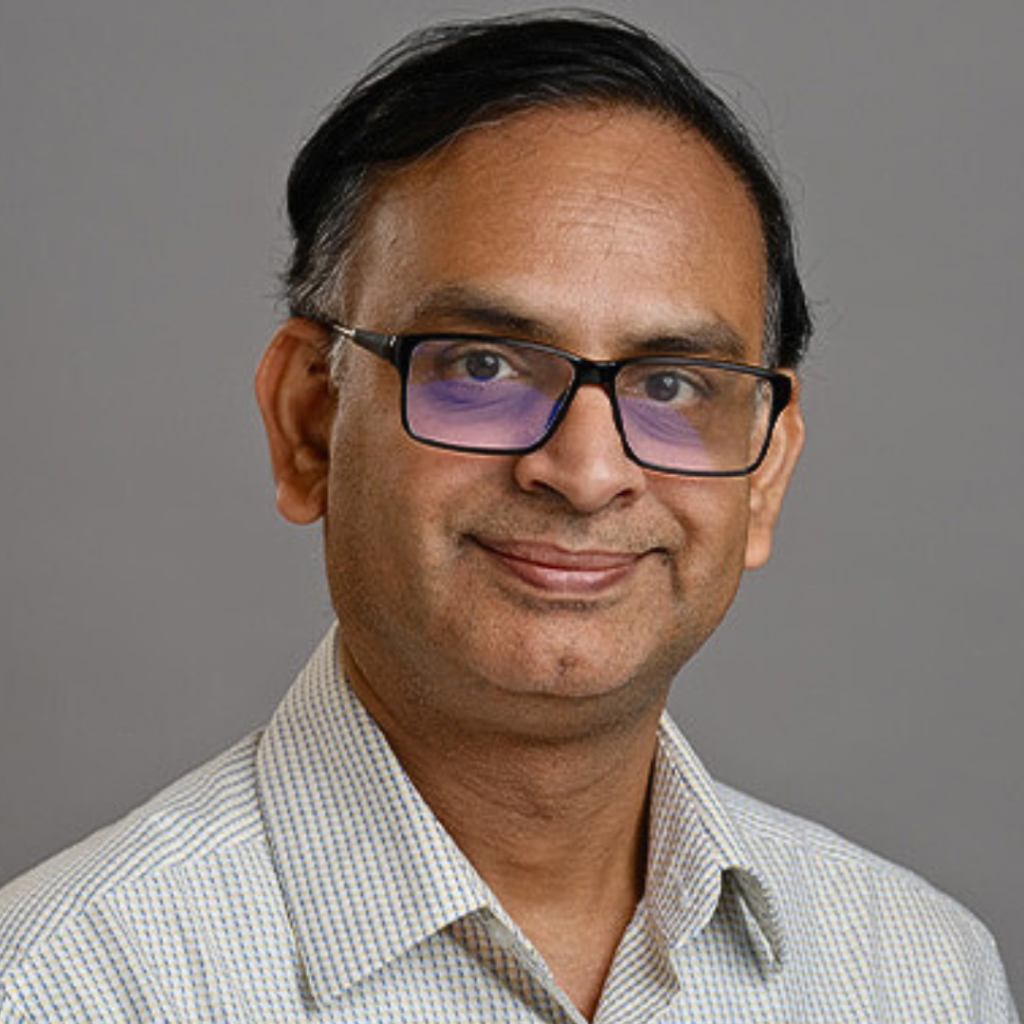
The Way forward for Medical Machine Expertise
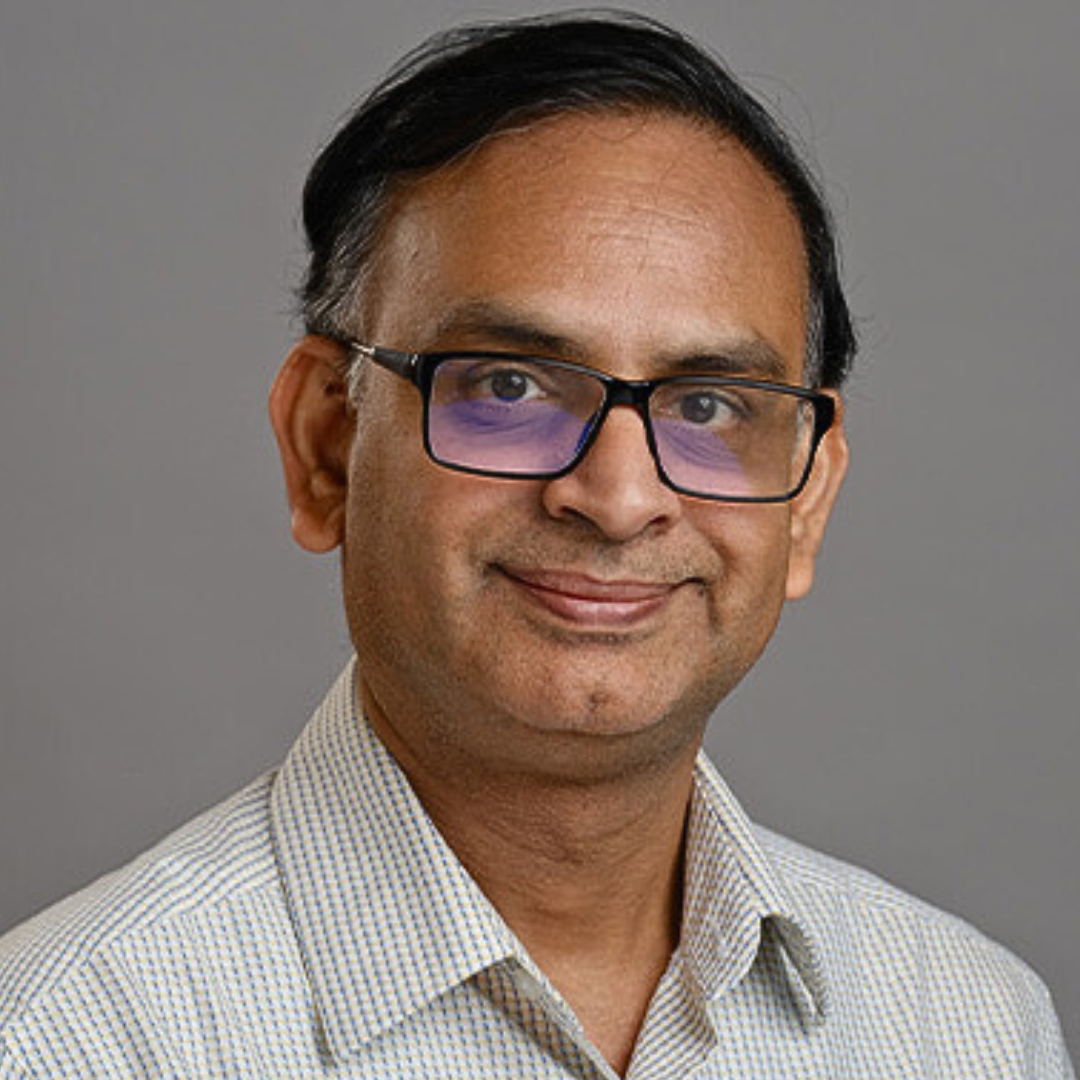
From predictive analytics to customized therapy plans, we’ve in all probability all heard in regards to the potential of AI to revolutionize medical prognosis and therapy. As mentioned in a latest NIH examine, “superior laptop algorithms have lately achieved accuracies akin to these of human specialists in medical sciences.”
However as AI algorithms are more and more built-in into medical gadgets and turn into extra influential in prognosis, making certain the security of the sufferers they work together with turns into more and more essential. Whereas these advances promise unprecedented precision and effectivity, in addition they introduce new complexities that have to be approached with nuance and foresight. We delve deeper into this crucial intersection of expertise and care, and discover how healthcare professionals can method these complexities—sustaining affected person security and privateness, relatively than compromising them.
An instance to reveal this shift comes from medical imaging. AI algorithms are being educated to research mammograms for breast most cancers detection, past what the human eye can detect. This might probably scale back the workload for radiologists, reduce human error, and enhance affected person care by enhancing early detection. Equally, AI-driven evaluation of retinal scans might determine diabetic retinopathy in its early phases, resulting in sooner motion and higher affected person outcomes.
It’s clear that using AI in medical imaging alone has grown exponentially. By the top of 2023, there have been almost 700 FDA-approved AI algorithms throughout varied healthcare specialties (up from simply 50 in 2013). 171 of those had been authorized between October 2022 and October 2023 alone. This speedy adoption is pushed by the promise of higher accuracy, effectivity and cost-effectiveness.
Medical gadgets with AI capabilities can rapidly analyze huge quantities of knowledge and supply real-time diagnostic assist. Monitoring gadgets that observe very important indicators and predict potential well being points earlier than they turn into crucial are understandably useful for efficient care. That’s why wearable applied sciences powered by AI can allow steady well being monitoring, alerting each sufferers and caregivers to abnormalities that require consideration. This can be a important step towards a extra proactive and customized method to healthcare, not simply in medical gadget innovation, however throughout all domains.
Challenges and obstacles
Nevertheless, this speedy adoption of AI expertise additionally brings distinctive challenges. As firms rush to capitalize on these groundbreaking capabilities, considerations have emerged over knowledge privateness, HIPAA compliance, knowledge bias, and the interpretability of AI algorithms.
Information privateness and HIPAA compliance are cornerstone elements that have to be constructed into any healthcare AI algorithm from the beginning. Whereas these algorithms depend on huge quantities of affected person knowledge to be taught and performance successfully, this knowledge might be anonymized and secured in accordance with HIPAA laws to forestall unauthorized entry and potential breaches. Reliability by way of safety, in addition to accuracy, uptime, and required upkeep are essential for adoption and belief, and must be rigorously examined by growth groups.
Bias is one other concern inside coaching datasets as it could possibly result in discriminatory outcomes. Coaching on sure knowledge that’s (even unintentionally) skewed towards a selected demographic can misdiagnose people from underrepresented teams. An extension of this transparency concern, the explainability of AI algorithms is equally essential. Healthcare professionals want to know the reasoning behind an AI-generated prognosis to make sure belief and inform their decision-making (in different phrases, these packages can’t be opaque “black field” algorithms). This goes again to the pillar of belief, as a result of with out clear insights into how AI methods make selections, healthcare suppliers might battle to belief and successfully use these applied sciences.
Significance of Danger Evaluation and High quality Assurance Practices
Danger evaluation frameworks provide one of the vital efficient strategies for navigating the complexities of integrating AI with medical gadgets and diagnostics. These methods emphasize the necessity for high-quality knowledge at each step, verifying that the data used to coach and validate AI algorithms is correct, full, and consultant of the goal inhabitants.
Interpretability, or the power to elucidate the reasoning behind an AI’s output, is one other essential side. By understanding the elements the algorithm thought of, healthcare professionals can assess its reliability and decide whether or not additional analysis or human experience is required. This goes past healthcare professionals’ analysis of diagnoses — regulators, healthcare establishments, and affected person households also can demand readability on how AI methods attain their conclusions.
Lastly, it’s important to deal with potential biases inside the knowledge and algorithms by means of QA practices comparable to rigorous knowledge cleansing, steady testing for rising biases, and extra. Danger evaluation frameworks ought to embody methods to determine and mitigate these biases and obtain truthful and equitable therapy for all sufferers.
Healthcare professionals should advocate for steady enchancment in threat evaluation practices and promote a tradition of affected person security above all else. Efficient testing (notably in areas comparable to biotech software program), probably incorporating automation for effectivity, have to be an integral a part of the event and implementation of AI-powered medical gadgets and diagnostics.
Presently, the promise of this unprecedented innovation is usually met with justified mistrust of present methods and suspicion of unexpected dangers. By prioritizing early (and steady) threat evaluation and implementing rigorous QA testing procedures, healthcare groups can keep on the forefront of AI in diagnostics and gadget innovation whereas upholding affected person security and moral issues. This paves the best way for a future the place AI serves as a strong software to enhance human experience and enhance affected person outcomes throughout the healthcare panorama, with out compromising affected person care or privateness.
About Dr. Sriram Rajagopalan
Dr. Sriram Rajagopalan is the Head of Coaching & Studying Providers and Enterprise Agile Evangelist at Inflectra, the place he designs coaching curricula and offers enterprise course of consulting. He’s additionally an adjunct school member at Northeastern College, the place he teaches programs on management, mission administration, Agile, and IT. Keen about youth management, Sriram based the Projecting Leaders Of Tomorrow (PLOT) initiative and wrote “Organized Widespread Sense” to assist it.