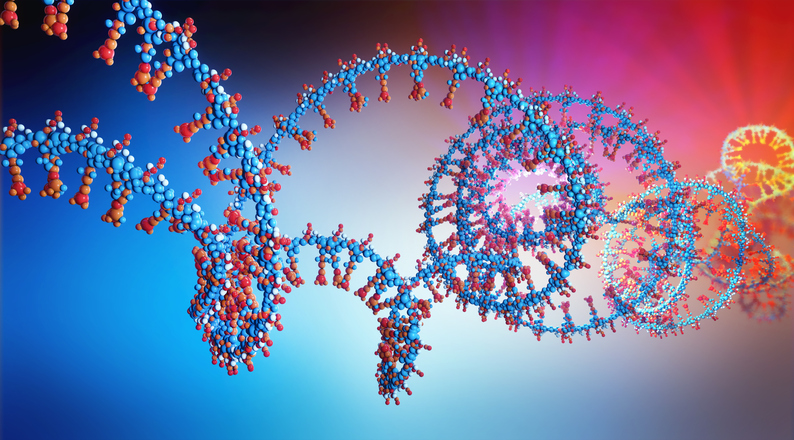
RNA Basis fashions to enhance productiveness in pharmaceutical analysis and improvement
It’s no shock that pharmaceutical analysis and improvement (R&D) stays one of many world’s most necessary hubs for innovation. Each pharmaceutical firm invests closely in and depends on R&D groups to create new therapies to assist the corporate develop and generate earnings. From 2012 to 2022, the business’s inflation-adjusted R&D spending elevated 44 p.c, from roughly $170 billion to $247 billion. Regardless of this funding, the variety of U.S. approvals of recent medication remained flat at a mean of 43 per yr. Productiveness challenges have come to the forefront as pharmaceutical firms attempt to leverage all obtainable sources.
Altering function of AI
As an business, we will now not tolerate vital productiveness points. A decade in the past, AI was promised as an answer to R&D productiveness, however to date this promise has fallen brief. We’ve got discovered the explanations for this shortfall, however extra importantly, there’s a new frontier of AI that addresses these causes and gives hope. The following frontier of AI is named ‘elementary fashions’ and they’re about to influence R&D. AI elementary fashions are like ‘ChatGPT for drug discovery’. Over the previous two years, elementary fashions related to pharmaceutical R&D have emerged and are on their approach to reworking the pharmaceutical business.
The primary decade of AI in biotech revolved round deep studying AI fashions that had been skilled to resolve particular duties utilizing task-specific information. For instance, a mannequin that predicts the results of gene modifying on splicing, one among dozens of organic processes essential to cell perform. Many educational labs and corporations, together with ours, confirmed that they may use these techniques to make correct predictions to help particular duties in drug discovery. Sadly, this method has typically fallen brief as a result of genomics, biology, physiology, therapeutics, and chemistry are advanced, multifaceted, and interconnected, so precisely accounting for only one process whereas leaving the various others untouched creates failure modes throughout R&D. Even when many various AI fashions are constructed for the totally different duties, they don’t seem to be skilled to account for the interrelatedness of the duties, and the complexity of getting many fashions makes scaling tough or unimaginable.
Basis fashions are a brand new method that modifications all that. They’re extraordinarily giant, deep-learning AI fashions skilled on large datasets that span a broad area and could be utilized to a variety of duties. They obtain synergies between these duties throughout coaching and when deployed in R&D purposes. They exhibit “emergent intelligence,” that means that they uncover and apply relationships and learnings that had been neither particularly included within the coaching information nor truly imagined by the creators of the AI. It’s not unusual for an AI basis mannequin to shock its creators by making predictions or revealing patterns outdoors of its supposed area of software. An instance of an AI basis mannequin outdoors of drug discovery is ChatGPT. AI basis fashions work as a result of they’re skilled on very giant and broad datasets, with billions and even trillions of information factors, which makes them versatile.
Decoding RNA biology to enhance R&D productiveness
As RNA biology and RNA therapies achieve traction within the pharmaceutical sector, RNA base fashions provide huge potential to revolutionize R&D.
RNA basis fashions can help R&D by predicting the results of affected person mutations, protein alterations, and therapeutic interventions on a variety of tissue-specific RNA organic processes, together with transcription, splicing, polyadenylation, protein-RNA interactions, and microRNA-RNA interactions. Therapeutic interventions can embody oligonucleotides, DNA modifying, RNA modifying, mRNA, and gene therapies. The method could be utilized to a variety of ailments and tissue areas, starting from widespread ailments to uncommon Mendelian problems to so-called 'N=1' therapies designed for particular person sufferers.
Curiously, know-how just isn’t the one issue. One of the essential components for productiveness enhancements is instilling a tradition of multilingualism, particularly on the subject of experimental biology and AI. Future success is dependent upon breaking down departmental silos and changing them with collaborations between computational scientists and biologists. These two various, interdependent groups should perceive one another to make sure open, efficient communication. Computational consultants should think about how every step might help discover extra molecules and targets, and biologists should pay attention to scale and information assortment.
The enterprise case for basis fashions
Now that this revolutionary TechBio know-how is accessible, pharmaceutical firms can use fundamental fashions to speed up innovation. Patents for a lot of high medication are expiring quickly, placing further strain on the TechBio business. For instance, Nova Nordisk’s patent on semaglutide, the energetic ingredient in each Wegovy and Ozempic, expires in China in 2026.
Based on McKinsey, many firms are prioritizing enhancing R&D productiveness and attempting to get extra worth from each greenback invested, given the looming patent expiration of blockbuster medication, a good pricing setting, and the prospect of pricing reform (significantly in, however not restricted to, the US).
Luckily for all pharmaceutical firms, fashionable advances in AI and biology are displaying the potential to speed up drug discovery, optimize scientific trials, and predict potential drug unintended effects. New AI foundational fashions, akin to these designed to decode RNA, are poised to enhance R&D productiveness, revolutionize drug discovery strategies, and guarantee a brighter future for pharma.
Photograph: Christoph Burgstedt, Getty Photos
Brendan Frey is a famend entrepreneur, engineer, and scientist, who’s the Chief Innovation Officer and Founding father of Deep Genomics. He’s a co-founder of the Vector Institute for Synthetic Intelligence and has made vital contributions to deep studying, genomic medication, and data know-how. Brendan has co-authored over 200 papers, a lot of which have appeared in prestigious scientific journals akin to Nature, Science, and Cell. His groundbreaking work on the “wake-sleep algorithm” with Geoffrey Hinton helped set up the sphere of deep studying. Brendan’s experience in AI led to the founding of Deep Genomics, the place he developed the primary AI system for predicting pathogenic mutations and therapeutic targets. He additionally holds positions on the College of Toronto and is a Fellow of a number of revered scientific societies. Brendan’s advisory roles at Microsoft Analysis and his intensive educational background additional show his influential contributions to the fields of AI and biomedical analysis.
This message seems through the MedCity influencers program. Anybody can publish their perspective on healthcare points and innovation on MedCity Information through MedCity Influencers. Click on right here to learn how.