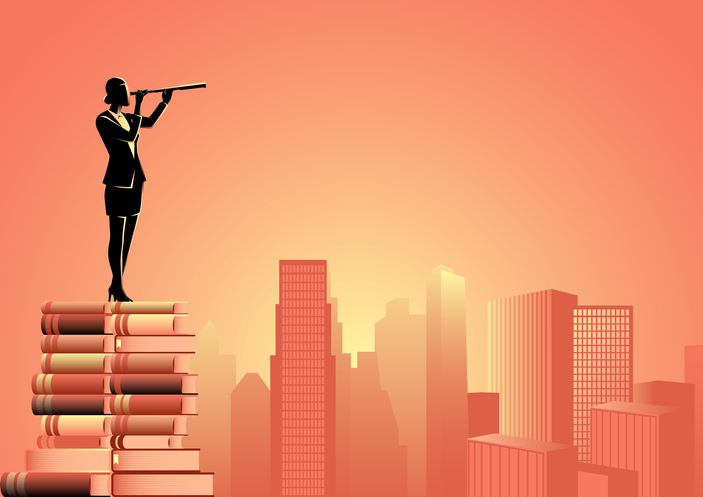
We're Overcomplicating AI in Healthcare – Easy methods to Obtain Impression Past the Hype
The early days of any foundational expertise are marked by overhype and skepticism. When the Web first burst onto the scene within the Nineties, fanatics have been awed by its potential to seek for virtually something on-line—even when nascent internet pages weren’t fully helpful—and imagined alternatives for a extra related world. Skeptics noticed the Web as a fad that will by no means change conventional strategies of communication or analysis. As time went on, there was a increase and bust—however ultimately, Web functions remodeled from cool novelties into sensible options to real-world issues. We’ve seen related cycles play out with cell telephones and social media. In the present day, we discover ourselves at an analogous crossroads for synthetic intelligence (AI) in healthcare.
When most individuals consider AI in healthcare, they envision futuristic functions, like “AI docs” serving to diagnose most cancers and AI brokers mechanically scanning your physique for cardiovascular occasions earlier than they occur. Whereas these improvements display the promise of AI for the long run, most have but to be deployed and validated at scale. If we wish to discover helpful AI functions in healthcare as we speak, we should look additional upstream to the place it may be transformative in fixing long-standing, complicated issues.
Scientific analysis is one such space ripe for the influence of AI. In the present day, knowledge administration continues to be a significant problem, and scientific researchers depend on guide processes to arrange and analyze massive quantities of healthcare knowledge. Whereas in most areas of our lives we’re used to typing queries right into a search engine and getting fast solutions, most scientific researchers nonetheless use spreadsheets to retailer, alternate, entry, and analyze knowledge. As the amount and complexity of multimodal healthcare knowledge has elevated with the rise of omics, the instruments we used to research them not work. Outdated processes result in delays in analysis and stagnation of innovation.
That is the place AI is available in – not with hype, however with an actual resolution to scientific researchers’ knowledge administration woes. AI techniques – significantly massive language fashions (LLMs) – can assist researchers set up knowledge into ready-to-use codecs and allow them to work with their knowledge extra effectively.
Let's take a deeper take a look at how these applied sciences are already enabling scientific breakthroughs in scientific analysis.
One space the place we see AI including worth as we speak is in accelerating knowledge harmonization. In its supply kind, scientific knowledge is messy. Researchers sometimes spend months sifting by way of digital silos and disparate knowledge codecs to harmonize info into a standard, research-ready construction. With the arrival of LLMs, researchers can pace up this course of 100x by way of AI-driven automation. LLMs harmonize mountains of knowledge from registries, analysis databases, and digital medical information (EMRs) right into a managed vocabulary prepared for evaluation. Downstream, researchers can skip the time-consuming knowledge wrangling and get straight to the science.
One other useful utility is extracting structured knowledge from beforehand unusable ‘unstructured’ knowledge, such because the free-text notes from an EMR. As a result of this info, which can comprise essential particulars a few affected person’s treatment use, for instance, just isn’t organized in a structured format, it’s typically excluded from analysis databases. LLMs can map this textual content from scientific notes into extra structured fields, answering questions resembling ‘did this affected person obtain radiation remedy’ or ‘did this particular person have a recurrence of most cancers’. This additionally works with pictures. For instance, we use AI-driven laptop imaginative and prescient to extract metadata from histopathology pictures, resembling cell counts, cell sorts, staining and extra. As soon as structured, all of this knowledge may be made out there for analysis, accelerating time to perception.
AI-driven person interfaces additionally radically enhance knowledge accessibility, creating a brand new paradigm for interactions between researchers and datasets. As an alternative of getting to be taught the technical complexities of databases—or collaborate with knowledge consultants who know tips on how to write code—AI-driven interfaces enable researchers to “chat with the info” utilizing pure language. As an alternative of writing a line of code, researchers can merely sort, “Discover me all sufferers who’ve had pathological stage II or greater breast most cancers and who’ve pathogenic variants on genes related to Lynch syndrome” into the AI-driven person interface. The LLM understands the request and converts it into a question in opposition to the harmonized dataset, returning a solution in seconds as an alternative of weeks. Not solely does this speed up time to perception, it additionally democratizes knowledge entry, opening the door for much more medical consultants to get entangled in analysis—particularly these with out programming expertise.
I consider that hype and skepticism are each good with regards to AI. These views will push the boundaries of what’s doable whereas bringing within the much less efficient use instances. However it’s essential that we give attention to the areas the place AI is already having a big influence. These are the sensible functions that enable the perfect and brightest researchers to give attention to science as an alternative of data-munching. Let’s get to that.
Picture: rudall30, Getty Photos
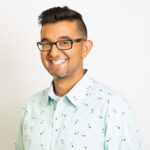
Sourav Dey is Chief Product Officer and Co-Founder at Manifold. He beforehand constructed AI/ML merchandise at Google. He was on the Nest AI/ML staff earlier than its acquisition by Google. His earlier work in algorithms and techniques engineering ranges from energy grid prediction at AutoGrid to wi-fi communications at Qualcomm. He holds patents for his work and has been revealed in a number of journals. Sourav obtained his PhD, MS, and BS in EECS from MIT.
This message seems through the MedCity influencers program. Anybody can publish their perspective on healthcare points and innovation on MedCity Information through MedCity Influencers. Click on right here to learn the way.