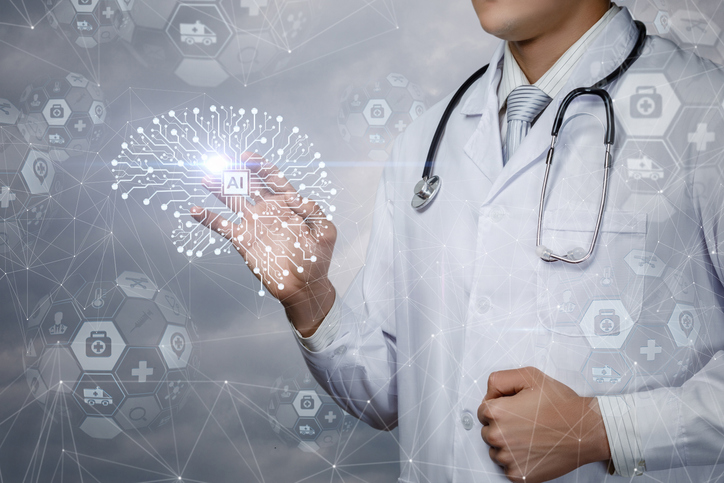
How AI can shut the hole in evidence-based care
Regardless that America is named a “melting pot” due to its numerous inhabitants, healthcare is highlighting a extra vital range difficulty. Healthcare is a extremely evidence-based observe that makes use of information in nearly each facet, from prognosis to therapy to testing of prescription drugs and medical units. But profound racial disparities have develop into the “norm” resulting from a scarcity of proof.
Solely 14 p.c of day by day medical choices are made primarily based on high-quality proof. That proof comes from 30 p.c of the U.S. inhabitants, as 70 p.c of the U.S. inhabitants is excluded from medical trials.
Though ladies make up half the inhabitants, ladies's well being care has traditionally been underfunded and researched. This can be partly as a result of FDA's 1977 coverage, which really helpful excluding ladies of childbearing age from early phases of drug trials. This finally led to a scarcity of knowledge on how medication can have an effect on ladies till a legislation was handed in 1994 requiring ladies's participation by the Nationwide Institutes of Well being.
Minority sufferers, together with those that are black, brown, and Asian, are additionally not often included in medical trials, creating vital proof gaps that end in lower than favorable outcomes or reinforcing stereotypes and biases primarily based on outdated, problematic algorithms that result in misdiagnosis and inappropriate diagnoses. remedies.
Black and American Indians and Alaska Natives (AIAN) have shorter life expectations, along with the best charges of pregnancy-related mortality. Native Hawaiian and Pacific Islanders, alternatively, are a inhabitants for which the well being care system has nonetheless not been capable of precisely analyze disparities as a result of there may be such a major information hole.
For instance, Native Hawaiians and Pacific Islanders make up lower than 0.2 p.c of Massachusetts' inhabitants. What would occur if somebody on this goal group was admitted to the emergency room and resisted the standard therapy plan for the native goal group? Sadly, this situation is extra widespread than you would possibly assume.
Conditions like these predispose sufferers to worsening situations until their care workforce has entry to bigger swimming pools of numerous information. On this instance, an ER in Boston would ideally pull information from a Hawaiian healthcare system to make sure the affected person receives probably the most acceptable and customized care, as demographics reply otherwise to therapy. Nevertheless, this observe can require hours of medical doctors sifting by analysis to find out what information is greatest for every affected person.
Minorities usually are not alone both. Rural communities are additionally misrepresented in proof gathering. As a consequence of a scarcity of entry and consciousness, solely a 3rd of medical trial contributors are from rural communities.
Rural healthcare programs are notoriously understaffed and under-resourced. Subsequently, offering medical doctors with information from city healthcare programs in the USA couldn’t solely generate extra constructive outcomes, however with AI, might additionally pace up care choices and provides medical doctors again priceless time that they might usually spend combing healthcare programs. by analysis.
Proof is vital to filling these gaps, and AI is required to translate proof into real-world insights.
Proof is the transactional unit of healthcare. That is how we determine which remedies to offer, measure the good thing about these remedies and make sure that we offer the best care to the best affected person. Given these disparities in entry to information, minority well being care lacks the proof wanted to tell these choices.
Healthcare and life sciences corporations should reevaluate their method to information and proof and fill information gaps with high-quality proof that may higher inform physicians and result in extra constructive affected person outcomes, no matter race, gender or location.
Thankfully, current developments in anonymously producing real-world proof and improvements in AI are permitting corporations to reevaluate current information units and bridge gaps with further outsourced information. By doing this, we will develop the accessible proof, making the dream of customized medication a actuality – even for underrepresented sufferers.
AI can present proof at scale, and never simply shortly. These instruments can run a whole bunch of 1000’s of research concurrently to generate complete proof for girls, kids and different demographic teams, equivalent to these with comorbidities and disease-based teams, which might be notoriously underrepresented in medical trials and different analysis.
One of many greatest obstacles to producing proof is the tedious evaluation of medical data and the prolonged de-identification course of. Generative AI exists to automate menacing duties, considered one of which is producing sensible proof. AI can pace up this time-consuming course of and supply researchers and clinicians with anonymized information that fills illustration gaps. These datasets can then be assembled and used to feed giant language fashions (LLMs) with extra correct proof of analysis high quality or to fill in lacking information for medical resolution making, making extra analysis accessible for minority populations.
Firms investing in LLMs wish to make sure that their mannequin's information is related and evidence-based. Correctly producing proof from revealed literature or real-world information can clear up this downside. Regardless of some physicians utilizing ChatGPT for medical resolution making, normal function LLMs like ChatGPT are unreliable in healthcare as a result of they aren’t fueled by real-world proof. The info it comes from shouldn’t be primarily based on real-world proof, producing inaccurate outcomes.
AI instruments are additionally designed to judge and enhance information high quality, permitting healthcare programs and life sciences corporations to determine gaps and take actionable steps to shut them in order that future healthcare choices are made with adequate proof. For physicians utilizing LLMs to tell choices, information analysis instruments can assess the standard of proof generated primarily based on how nicely it matches a affected person's background. It may possibly additionally generate future care recommendations and report up to date information for later use. Information analysis instruments may even inform clinicians how nicely a supplied information set suits their query, revealing the reliability of recommendations and uncovering any inconsistencies within the solutions.
AI will allow us to offer extra personalization in healthcare. With proof generated in minutes, the times of broad tips might be over as researchers and clinicians may have customized proof at their fingertips that may remodel value-based care.
As we method 2025, AI instruments should concentrate on producing high-quality proof. Those that do that by transparency, high-quality methodology, and the flexibility to get a trust-based response from physicians would be the ones to achieve the long run and remodel evidence-based care.
Picture: Natali_Mis, Getty Photos
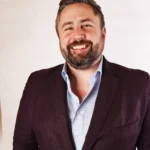
Dr. Brigham Hyde has been CEO and co-founder of Atropos Well being since August 2022. Hyde has a major monitor report of constructing corporations in well being know-how and real-world information (RWD) and most lately served as President of Information & Analytics at Eversana. Previous to that function, Mr. Hyde was a healthcare companion at AI enterprise fund Symphony AI, the place he led the funding in Live performance AI, an oncology RWD firm lately valued at $1.9 billion, and co-founded and was the operator. Hyde beforehand held positions as Chief Information Officer at Determination Assets Group, which was acquired by Clarivate for $900 million in 2020. He additionally served on the World Information Science Advisory Board for Janssen, as a analysis college member at MIT Media Lab, and as a analysis college member at MIT Media Lab. an adjunct college member at Tufts Medical College.
This message seems through the MedCity Influencers program. Anybody can publish their views on enterprise and innovation in healthcare on MedCity Information through MedCity Influencers. Click on right here to see how.