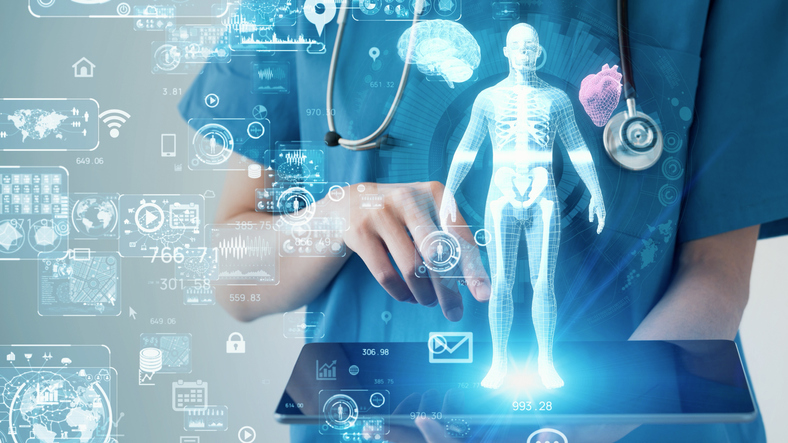
On this spherical: People 1, AI LLMs 0
A brand new research that pitted six people, OpenAI's GPT-4 and Anthropic's Claude3-Opus, towards one another to judge which ones may reply medical questions most precisely discovered that flesh and blood nonetheless outperforms synthetic intelligence.
Each LLMs answered a couple of third of the questions incorrectly, though GPT-4 carried out worse than Claude3-Opus. The survey questionnaire was primarily based on goal medical information from a Information Graph created by one other AI firm, Israel-based Kahun. The corporate created its personal Information Graph with a structured illustration of scientific details from peer-reviewed sources, in accordance with a press launch.
To organize GPT-4 and Claude3-Opus, 105,000 evidence-based medical questions and solutions had been fed into every LLM from the Kahun Information Graph. This contains greater than 30 million evidence-based medical insights from peer-reviewed medical publications and sources, the corporate stated. The medical questions and solutions (QAs) fed into every LLM spanned many alternative healthcare disciplines and had been categorized as both numerical or semantic questions. The six individuals who answered the questionnaire had been two physicians and 4 medical college students (of their scientific years). To validate the benchmark, 100 numerical questions (questionnaire) had been randomly chosen.
It seems that GPT-4 answered almost half of the questions with numerical solutions incorrectly. In response to the press launch, “Numerical QAs take care of correlating findings from a single supply for a particular question (e.g., prevalence of dysuria in feminine sufferers with urinary tract infections), whereas semantic QAs take care of differentiating entities in particular medical queries (e.g., choosing the most typical subtypes of dementia). Critically, Kahun led the analysis group in laying the groundwork for evidence-based QAs that resembled brief, single-item queries {that a} doctor would possibly ask themselves in on a regular basis medical decision-making processes.”
That is how Kahun's CEO responded to the findings.
“Whereas it was fascinating to notice that Claude3 was higher than GPT-4, our analysis reveals that normal LLMs nonetheless don’t outperform medical professionals in deciphering and analyzing medical questions a doctor encounters each day,” stated Dr. Michal Tzuchman Katz, CEO and co-founder of Kahun.
After analyzing over 24,500 QA responses, the analysis group found these key findings. The press launch notes:
- Claude3 and GPT-4 each carried out higher on semantic QAs (68.7 and 68.4 %, respectively) than on numerical QAs (63.7 and 56.7 %, respectively), with Claude3 outperforming on numerical accuracy.
- The analysis reveals that every LLM generates completely different outcomes on every project. This highlights the significance of the truth that the identical QA project can yield vastly completely different outcomes between completely different fashions.
- For validation, six medical professionals answered 100 numerical QAs and outperformed each LLMs with 82.3 % accuracy. Claude3 had 64.3 % accuracy and GPT-4 had 55.8 % accuracy when answering the identical questions.
- Kahun's analysis reveals how each Claude3 and GPT-4 excel at semantic queries, however in the end helps the proposition that normal LLMs aren’t but effectively outfitted to be a dependable data assistant for physicians in a scientific setting.
- The research included an “I don’t know” choice to mirror conditions the place a doctor should admit uncertainty. It discovered completely different response charges for every LLM (Numeric: Claude3-63.66%, GPT-4-96.4%; Semantic: Claude3-94.62%, GPT-4-98.31%). Nonetheless, there was an insignificant correlation between accuracy and response fee for each LLMs, suggesting that their capability to confess lack of know-how is questionable. This means that with out prior information of the medical subject and the mannequin, the reliability of LLMs is questionable.
An instance of a query that individuals answered extra precisely than their LLM colleagues was this: “What’s the prevalence of sufferers with fistulas amongst sufferers with diverticulitis? Select the proper reply from the next choices, with out including any additional textual content: (1) Larger than 54%, (2) Between 5% and 54%, (3) Lower than 5%, (4) I don't know (provided that you don't know what the reply is).”
All the physicians/college students answered the query accurately, and each fashions had been fallacious. Katz famous that the general outcomes don’t imply that LLMs can’t be used to reply scientific questions. As an alternative, they need to “embrace verified and domain-specific sources of their knowledge.”
“We’re excited to proceed contributing to the development of AI in healthcare via our analysis and by offering an answer that delivers the transparency and proof important to help physicians in making medical selections,” he added.
Kahun desires to construct an “explainable AI” engine to debunk the notion many have of LLMs — that they’re largely black bins and nobody is aware of how they arrive to a prediction or resolution/advice. In truth, 89% of physicians in a current April survey stated they should know what content material LLMs used to succeed in their conclusions. That stage of transparency is prone to drive adoption.
Photograph: metamorworks, Getty Pictures